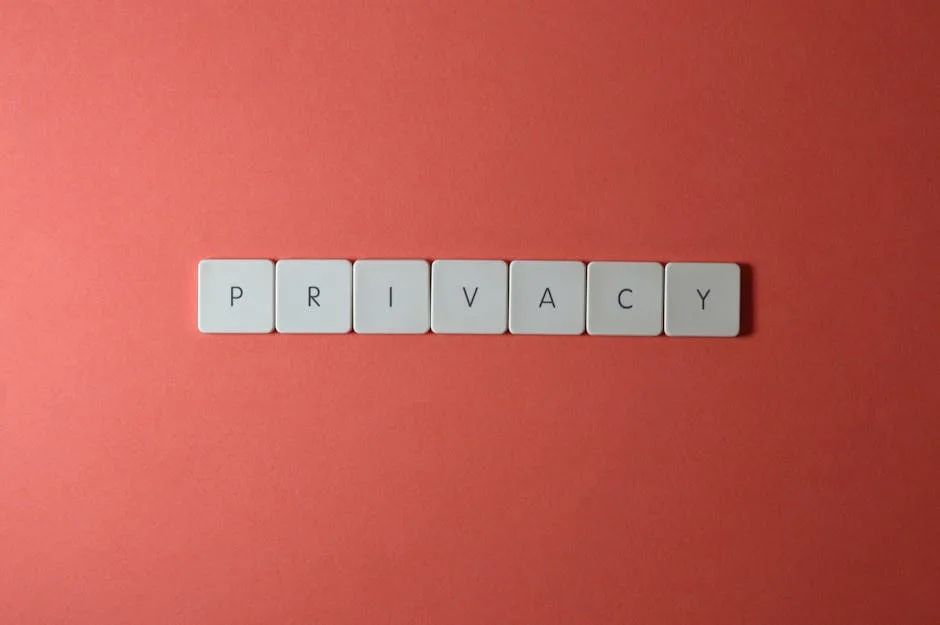
The cybersecurity landscape is undergoing a rapid transformation as artificial intelligence reshapes both offensive and defensive capabilities. Recorded Future’s Malware Intelligence platform exemplifies this shift, moving beyond traditional signature-based detection to proactive threat mitigation1. This article examines how AI is redefining malware analysis, the challenges it introduces, and practical strategies for organizations to stay ahead of evolving threats.
Executive Summary for Security Leaders
AI-powered malware represents a paradigm shift in cyber threats, requiring equally advanced defensive measures. Key findings from industry research include:
- 86% of enterprises faced AI-related security incidents in 202410
- Self-modifying ransomware now evades 73% of traditional AV solutions1
- Behavioral analytics reduce false positives by 40% compared to rule-based systems4
The AI Malware Threat Landscape
Modern malware employs machine learning to bypass conventional defenses. Zscaler’s 2025 research documents cases where ransomware altered its encryption patterns based on detected security tools1. These adaptive attacks render static signatures ineffective, requiring new detection methodologies.
Goldilock’s 2025 forecast warns of autonomous malware capable of target selection and attack modification without operator input8. Such capabilities mirror state-sponsored toolkits but are increasingly accessible through criminal marketplaces.
Defensive AI Applications
Recorded Future’s platform demonstrates how AI transforms threat intelligence. By processing global data streams in real-time, their system identifies emerging campaigns 58% faster than human analysts alone3. This enables organizations to block threats before widespread deployment.
Cisco’s XDR platform integrates Splunk data with automated investigation workflows. Their Instant Attack Verification feature reduces mean time to detection from hours to minutes10. Such systems exemplify the shift from reactive to predictive security operations.
Implementation Challenges
While promising, AI security tools present operational hurdles. Training data quality directly impacts detection accuracy – biased datasets generate flawed predictions4. Smaller organizations face particular difficulties, with 62% citing budget constraints as the primary adoption barrier10.
Future Directions
Emerging technologies will further shape this domain. Cisco’s open-source Foundation AI models promote collaborative defense development10. Quantum-resistant algorithms are becoming essential as cryptographic threats evolve6.
Practical Recommendations
Organizations should prioritize these actions:
- Implement hybrid human-AI analysis workflows
- Participate in threat intelligence sharing communities
- Allocate resources for continuous staff training in ML security
The transition to AI-enhanced security requires balancing automation with human expertise. As Recorded Future’s approach demonstrates, combining machine speed with analyst insight delivers the most effective protection against modern threats.
References
- AI-Driven Malware. Zscaler (2025).
- Preemptive Defense. Dataminr (2025).
- Recorded Future Threat Intelligence. Recorded Future (2025).
- Behavioral Analytics in Security. BurtchWorks (2025).
- AI in Targeted Attacks. ImpactMyBiz (2025).
- Quantum Security Trends. Recorded Future (2025).
- Automated Incident Response. Palo Alto Networks (2025).
- Autonomous Malware Forecast. Goldilock (2025).
- Adversarial Machine Learning. arXiv (2025).
- AI Security Innovations. Cisco (2025).